Artificial neuromodulator-synapse array
reduces energy consumption by up to 75% compared to conventional CNN deep learning
Prof. Wang Gun-uk & and Prof. Jang Jingon publish results in Nano Energy
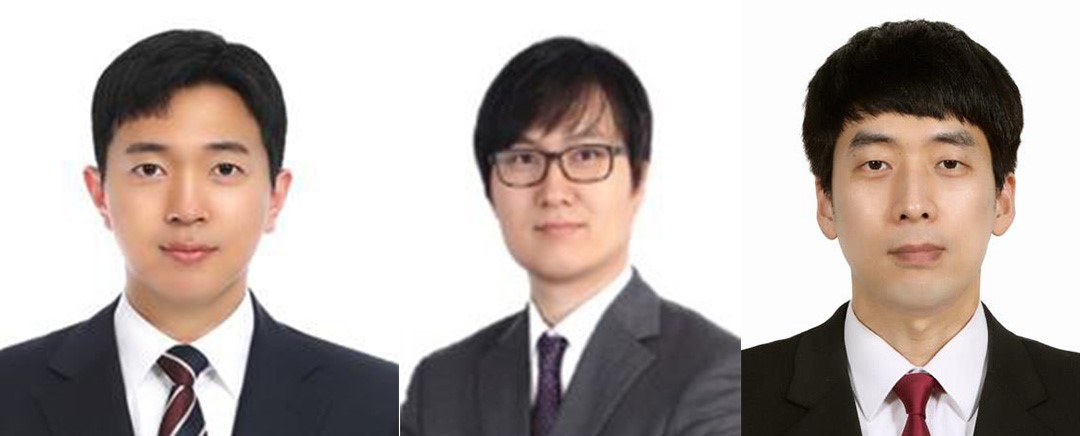
▲ (From left) Dr. Ham Seong-gil of KU-KIST Graduate School of Converging Science and Technology, Prof. Wang Gun-uk of KU-KIST Graduate School of Converging Science and Technology, Prof. Jang Jin-gon of Kwangwoon University’s School of Computer and Information Engineering
The team led by Prof. Wang Gun-uk of the Department of Integrative Energy Engineering at the KU-KIST Graduate School of Converging Science and Technology, along with the team under Prof. Jang Jin-gon of Kwangwoon University’s School of Computer and Information Engineering, proposed a new hardware platform capable of fast and energy-efficient convolution image processing through the implementation of an artificial neuromodulator-synapse (memristor) array device with a three-terminal diagonal gate structure.
The research findings were published online on March 15, 2024, in the prestigious interdisciplinary journal Nano Energy (IF=17.6).
- Title of paper: Artificial neuromodulator–synapse mimicked by a three-terminal vertical organic ferroelectric barristor for fast and energy-efficient neuromorphic computing
- Author information: Ham Seong-gil (first author, KU-KIST Graduate School of Converging Science and Technology), Jang Jin-gon (first author, School of Computer and Information Engineering, Kwangwoon University), Wang Gun-uk (corresponding author, Department of Integrative Energy Engineering, KU-KIST Graduate School of Converging Science and Technology)
Recently, image data processing has become increasingly important in big data applications such as object recognition, image processing, computer vision, autonomous driving, security, and medical analysis.
One method of processing such image data in hardware is neuromorphic computing based on next-generation non-volatile resistance change memory devices. However, the efficiency of repetitive vector-matrix multiplication operations between input signals such as images and memory device weighting information has yet to be improved. As a possible solution, research is being conducted into the development of composite array devices with three-terminal structures instead of conventional two-terminal memristor crossbar array structures.
Although conventional passive crossbar arrays are useful for parallel data processing through composite input voltage information, as the convolution area and kernel operation count increase, the increase in the number of kernel sliding operations in the image scan area leads to increased data processing delays and significant energy consumption due to higher intermediate neuron data storage requirements.
<Fig 1>
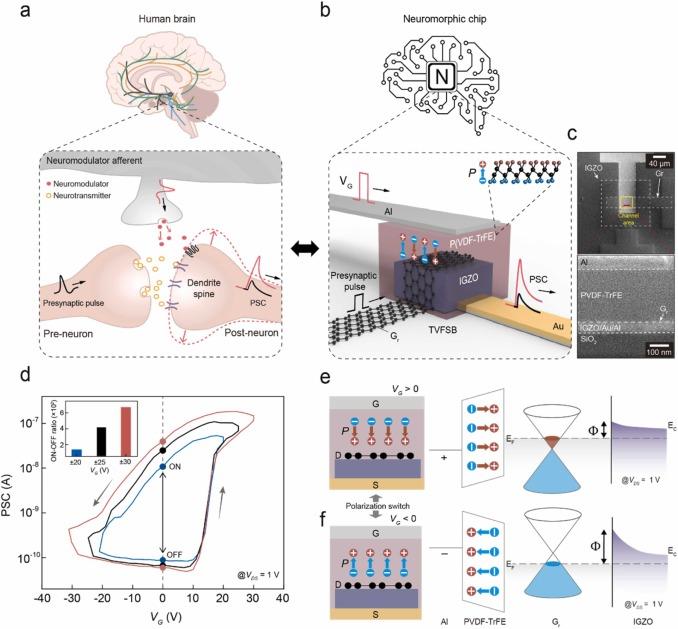
▲ Analysis of the three-terminal diagonal gate memristor device structure and synaptic plasticity induction mechanism
The team developed a hardware system implementing simultaneous weight learning of shared gate lines using a three-terminal diagonal memristor device. The proposed array device platform, designed for fast and convenient convolution transformation operations, can extract features from neighboring convolution transformation areas, reducing the dimensions of feature maps through pooling.
In applications involving massive-scale image and video processing, this one-step convolution transformation allows pooling operations to be performed directly and without delay, enabling the faster and more energy-efficient processing of image operations that need to be carried out within a specific time frame. This solution expected to provide a groundbreaking direction for computer vision applications utilizing hardware computation.
<Fig 2>
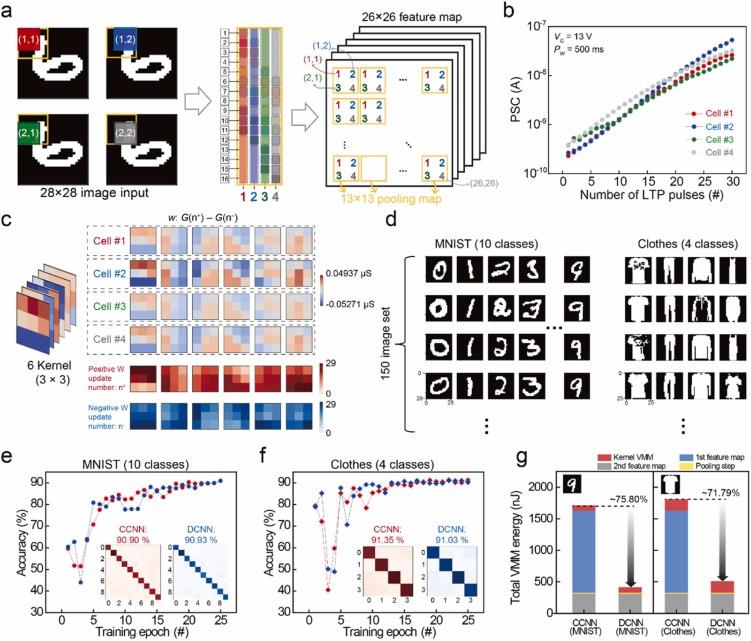
▲ Convolution image processing using a three-terminal diagonal gate memristor array device. Unlike the conventional method of sequentially performing vector-matrix multiplication operations between the kernel area and partial image area, this process performs convolution operations on the expanded image area simultaneously. Images are directly transformed into a pooling map without intermediate data storage, directly converting to analog pooling, thereby reducing energy consumption by approximately 75% and leading to faster processing than with existing techniques.
The study was supported by the National Research Foundation of Korea’s Experienced Researcher Program and Nanomaterial Technology Development Program, KIST Institutional Program, and the Ministry of Education’s Creative & Challenging Research Program.